Big Data maturity measures the level of a company’s use of data. The more complex their use of data, the higher the Big Data maturity level. We have previously written about Big Data maturity levels, so you can check it out to learn about it.
But we know another Data science expert who has put his knowledge about Big Data (Science) maturity levels into comprehensible words in his article on Vespa’s blog and he is Jon Bratseth.
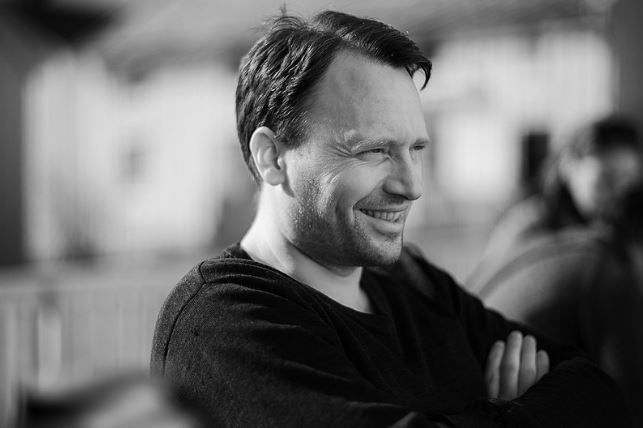
Image Source: Jon Bratseth
Jon is a VP architect in the Big Data and AI group of Verizon Media, and the architect and one of the main contributors to Vespa.ai, the open Big Data serving engine.
With 20 years of experience as architect and programmer on large distributed systems, as well as a frequent public speaker, Jon has spoken about Big Data serving, with a special mention of Big Data maturity, in his presentation at the Big Data Conference in 2019.
In case you haven’t heard, Big Data serving refers to the “selection, organisation and machine-learned model inference involving constantly changing Big Data sets with low latency and high load”. It is an example of the highest Big Data maturity level, Acting out of learned data patterns.
You can watch his presentation to learn more.
On that note, we decided to reach out to him with questions about data maturity and Big Data serving. Without further ado, here’s what Jon had to say:
[Iunera] Why do different sources give different sets of data maturity levels? Wouldn’t this lack of standard be confusing for Big Data maturity assessments?
[Jon] Yes, fields that are developing, where new ideas, techniques and solutions keep appearing, can be confusing.For something to be standardized, many people need to agree to the content of the standard, and achieving that wide agreement takes a long time. It cannot happen in fields that are still in rapid development.
This is why the saying among people who like rapidly developing fields is: Only that which is no longer interesting can be standardized.
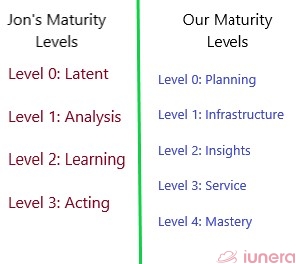
[Iunera] Besides movie streaming, what other examples can demonstrate Big Data maturity levels?
[Jon] Another example could be a credit card company who wants to detect fraud.At first they just produce a log of transactions (latent).
Then they hire humans to analyze the transaction log data to highlight unusual patterns of transactions which should be investigated by humans (analysis).
Then they automate this process by using machine learning to automatically find fraudulent transactions (learning).
Lastly, they move to blocking fraudulent transactions as they are attempted (acting).
[Iunera] How would you define latency to someone who has never heard of it before?
[Jon] It just means how long it takes something to react to something you are doing.For example, if you are clicking a button on your phone, how long does it take until you see the result. Or to continue the credit card example, if you are in a store making a transaction, how long you have to wait until it goes through.
[Iunera] Can Big Data serving be used for essential fields like medical, legal, transport, agriculture, energy and so on? If yes, how?
[Jon] So far, it’s mostly the largest internet companies that have reached level four, where they act in real time on the basis of data.I believe the main reason this hasn’t yet penetrated to other industries is that it has simply been too hard to build the required technology. This changed with Vespa – a big data serving platform used on some of the largest internet use cases in the world – becoming open source.
On a high level it seems obvious that using data to act in real time, where you have the most up to date and detailed knowledge to make a decision, [is helpful] but people working in each industry ultimately need to do the innovation work to apply this to their specific problems.
We believe the next industry after internet/media that will make a large move into level four is finance, because it is relatively technologically advanced already, and because the payoffs are so large. We’re already seeing companies working on some very interesting use cases here.
[Iunera] In terms of serving algorithms, what kind of applications do you view as especially valuable besides the collaborative filtering (recommendations)?
[Jon] The first use case of big data serving, which funded its development, was web search. Web search, search and information exploration in general is still a huge and growing field.The next use case of big data serving we have seen grow to maturity is recommendation, personalization, and ad targeting. This already represents value on the order of hundreds of billions of dollars a year and its transformation to big data serving is very far from complete.
What use case will be next to have an impact on this level? One of the most relevant candidates is probably finance.
What we can be sure of is that just as in the earlier waves those who find these solutions first will stand to gain a lot, and that the large technological barrier that existed in the previous waves have now been lifted.
[Iunera] Do you have an example for the current Covid-19 situation? If yes, then how would Big Data serving advance the situation?

As an example of the former, my team created CORD-19 Search, a Vespa application providing advanced search and exploration capabilities in the body of scientific knowledge relevant to Covid-19, to aid researchers working in this space find the information they need.
This goes beyond traditional search by embedding articles in semantic vector spaces to allow exploration of a topic by following trails of semantic relatedness between articles.
As an example of the latter, a team used Vespa to quickly create a service providing real time information on mask availability near your location as a way to mitigate mask supply shortages during the pandemic.
[Iunera] How do you see Big Data serving in relation to online learning?
[Jon] Online learning requires two things: The learner must be able to work incrementally, and the serving system applying the model must make it possible and efficient to update model parameters incrementally.In Vespa, we ensure the latter, while the former is left to the subsystem doing the learning. Here’s a case study which shares how a particular online learning system was implemented on Vespa.
[Iunera] What do you see as the main obstacles to reaching the Acting level of big data serving?
[Jon] Up until recently, it has been the very high cost, time and skill level required to develop the requisite technology.This changed with Vespa becoming open source, as it provides everyone access to a mature technology stack for big data serving.
Now I believe it is dissemination and absorption of the idea and the availability of the technology.
[Iunera] What is the best practice to deal, monitor and react to concept drifts when serving models?
[Jon] The best way to combat concept drift is to update and retrain often. Vespa makes it easy to update both models, and individual model parameters, however, this also requires streamlining the learning side as the actual learning happens outside Vespa – a larger topic.[Iunera] What value do you see in the Latent, Analysis and Learning levels (the levels before the Big Data serving level)? Is there an economic return that can be quantified before you ultimately act on the data?
[Jon] Yes, each progression brings large economic returns, stemming generally from improved decision making, with less human labor, and – depending on specifics – enabling entirely new solutions.