There are different ways how Big Data Analytics and Smartphones help to contain the Coronavirus pandemic. This is especially with the Time Series Analysis which can support different cases to break the Coronavirus infection chains. Thereby, Big Data and privacy challenges go hand in hand. Hence, we compare the top 5 ways how smartphones and Big Data Analytics can help to contain the Coronavirus pandemic and to break infection chains.
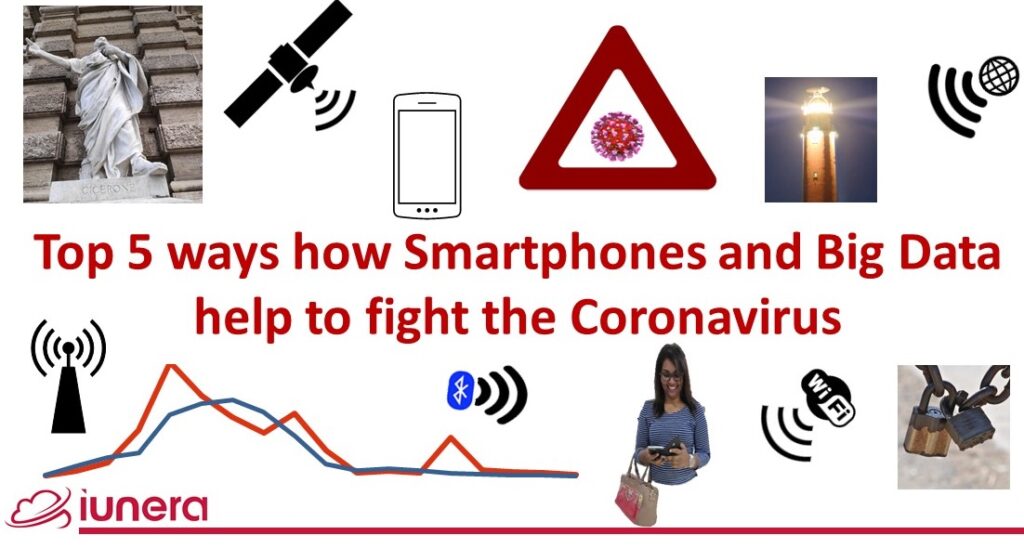
First, we discuss how mobile operator signals can be anlayzed with Big Data.
After that, we focus on how privacy-compliant Coronavirus tracking with a Bluetooth app and a distributed backend can be implemented.
Subsequently, we provide complete insights for Coronavirus geotracking with a special app and provide details on how Coronavirus geotracking can be more anonymized by location fingerprinting.
Finally, we will show opportunities in using third party data for analysis and give a conclusion. Therefore, we will show a comparison matrix between the different approaches.
Mobile provider Big Data signal analytics
In another article, we will discuss in detail how Mobile network provider data analysis with Time Series works. Here we will provide a brief overview about the essence.
Social distancing and movement restrictions are the only way to slow down the Coronavrius spread until vaccines and treatmeants for Covid-19 are available.
Therefore, governments of the world aim to slow down the Coronavirus spread by introducing movement restrictions.
Decisions based purely on the Coronavirus infection rate are always delayed due to the incubation time which makes it difficult to see if the movement restrictions or controls were even obeyed.
Why?
Mobile provider Big Data analysis can help to control, in a coarse grained and anonymous way, if movement and contact restriction orders are changing behaviours.
One way for optimization is to use the provider data for mobile Big Data analysis.
How does it work?
Mobile phones connect to a primary cell tower and know other towers near this tower.
Once a user moves into a new area a handover to a new celltower is done. Different cell towers and their signal strength reception can be used for coarse grained location triangulation.
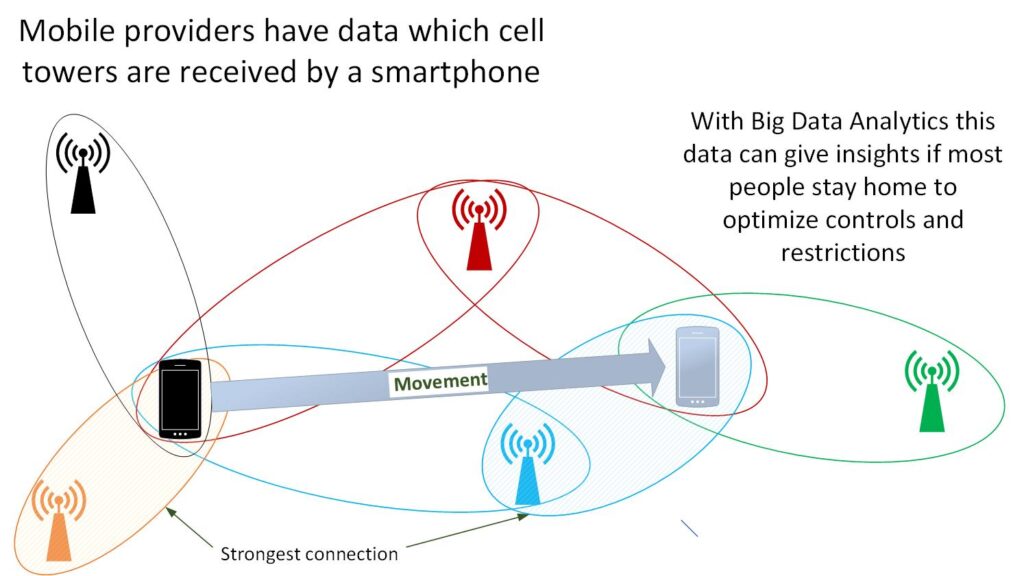
What is required for this?
In order to do these Big Data, a map of cell towers and time series data from mobile network operators is needed. These time series data then needs to be processed and analyzed with Big Data tools.
How preceise and privacy compliant is it?
It is a coarse grained method.
When the identifiers of sole users are replaced on a daily foundation in the big dataset it will be very unlikely to indentify sole users easily.
In addition to that, the triangulation capabilities are coarse grained, which fuzzyfies the locations of users naturally.
Can it break infection chains?
Big Data dashboards can show coarse grained problem zones. There one can see where people move most. In addition, those areas can then be correlated with infections.
However, this Big Data analysis method is not precise and is rather a “geo-regional infection chain breaker” rather than a concrete infection chain breaker of individuals.
All in all, the infection chain breaking capability is coarse grained.
Privacy compliant Bluetooth tracking
In a previous article, we discussed in detail how privacy compliant Coronavirus tracking with Bluetooth and Blockchain works. Here we give a brief overview of the essence.
Mobile provider tracking is coarse grained. Concrete fine grained privacy compliant tracking can be done by short distance communication with Bluetooth.
Why?
Coarse grained location data is not able to help to find potentially infected people.
Therefore, an approach is needed which matches people who finds out that they are infected with people who they had contact with in the past.
Privacy and security can be the decision factor for users to install an app which deals with such crucial health details.
Acceptance of such a Coronavirus app is vital, because it needs 60% of an population to work.
Therefore, short distance communication in the form of Bluetooth can help to achieve the privacy goals where people exchange these crucial information directly.
How it works
Users emit regularly signals with their anoymous identity.
Once two people meet, they receive their identities and some kryptographic additions and store hash values of their meeting locally on their phones.
These hash values cannot be resolved to the original exchanged values which makes it impossible for third parties to know which data was exchanged.
Once a person is infected the hash values receives signed signs on them with the secret identity key which the partner with whom the data was exchanged previously knows.
Then the signed data is uploaded into an online resource where the pairing partners can download the records and determine their infection risk.

As backend sharing the infection record, a Blockchain can be advantagous to avoid potential corruption, flooding it with fictitious values and ensuring transparency and no single party control.
Furthermore, a Blockchain can help anonymity; imagine the users emit stealth addresses of privacy focused Blockchains to pairing partners and later on when one of the recipients is infected a transaction with infection metadata is sent to this stealth address.
What is required for applying this?
A smartphone Coronavirus tracking app is capable of broadcasting and receiving Bluetooth signals. Furthermore, the app needs to be able to maintain a local database about emitting and receiving signals.
As a data exchange backend, an infrastrcuture is needed where a user can proof their authenticity to the people whom he/she meet. Furthermore, the backend shall be distributed and tamper proof to protect from spamming attacks.
How precise and privacy compliant is it?
The level of privacy for this method is high.
One possible attack is the personal memory of a participant to remember who he have met and therefore who might have been a carrier when an infection occurs.
When implemented in a secure ware, the main remaining and possible attacks are by capturing of the metadata transfer or compromising the backend what can be minimized by using cryptography and a transparent backend.
All in all, the technique is highly secure, but clearly not perfect.
Can it break infection chains?
People who have had contact with an infected person can be notified about a potential Coronavirus infection. This makes it possible for these people to recognize their infection faster and the infection chains can then be broken.
However, there is an incubation time of the Coronavirus so the potentially infected people might already have infected new people when they are notified.
Since everything is anoymous, this second level potential Coronavirus infections cannot be notified with this approach.
Extended geolocation tracking
In another article, we discussed completely how extended location tracking with GPS, WiFi and Bluetooth works. Here we give a brief overview about the essence.
In contrast to privacy focused apps with Bluetooth short distance communication, apps which are tracking concrete geolocation data can help to gain more Big Data analysis insights.
Why?
Special Coronavirus geotracking apps open possibilities to geotrack possible infections in a much more precise manner.
In addition, they allow for understanding of infection chains, also from a fine grained geographic perspective.
How it works
A smartphone app shares its location continously with a Big Data tracking server or stores it locally.
Once a Coronavirus infection occurs, the geolocations of the past of the infected person can be matched with other people.
In order to do such a matching easily, Big Data technology like Time Series Databases can be used.
The matching analysis reveals users who have been longer than 15 meters in close proximity to the Coronavirus infected person. They then can be warned in sufficient time in advance and advised to take precautions not to infect others.
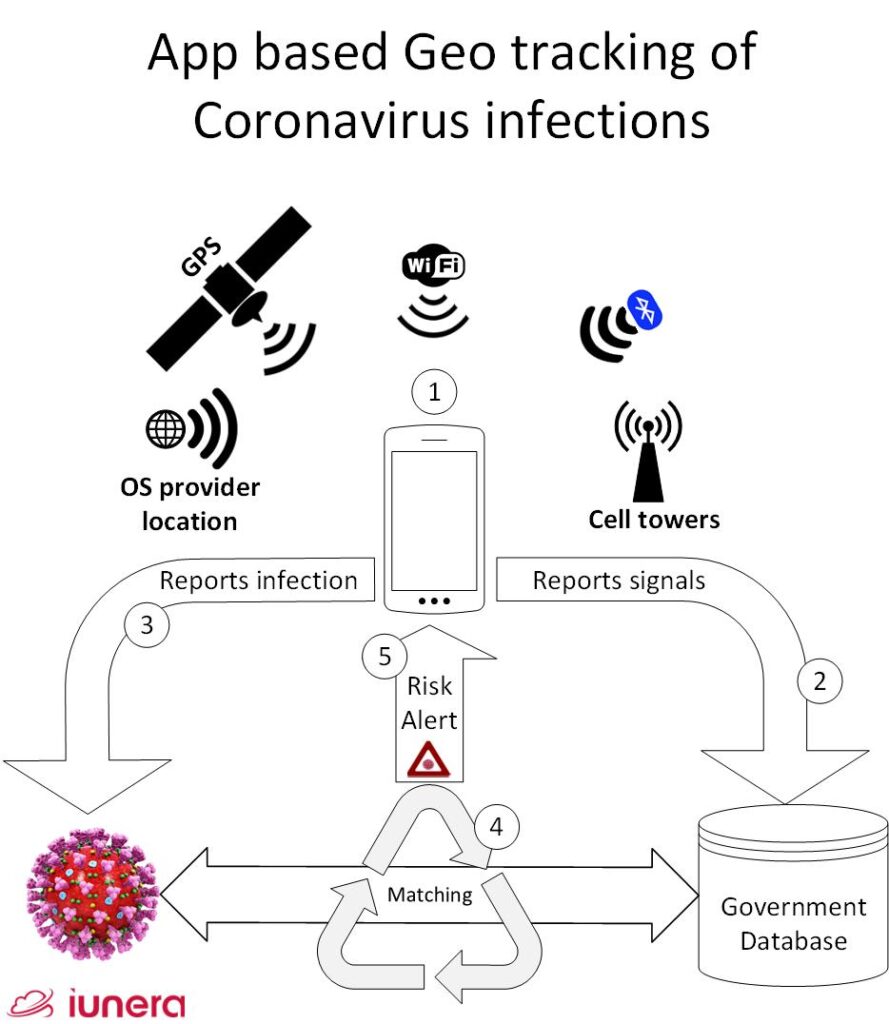
What is required to do it?
A special app is needed when recording regularly telemetry data where a person was in the background.
Also, a Big Data database server is needed where the time series of people’s movements is matched.
How preceise and privacy compliant is it?
The price of this method is that time based habits of users can be analyzed.
For instance, an analysis can be done how long an infected person have been in one place and extrapolate their life, workplace and leisure activities.
Therefore, this method is not very privacy compliant.
Can it break infection chains?
This method has an immense potential for breaking infection chains.
When this method is combined with Coronavrius test results, it can likely be used to train machine learning to even predict infection chains.
Location fingerprint tracking
In another article, we discussed in detail how fingerprints can be computed on top of extended location tracking. Here we give a brief overview about the essence.
Location fingerprint tracking is a privacy refinement version of the extended Coronavirus location tracking.
Why?
The previously described extended location tracking transmits a lot of data about a user.
Looking at the sole case of matching locations where two people have been at the same time, the question arises if we can come up with a method that delivers similar Coronavirus tracking results, but with more privacy.
How it works
WiFi networks, Bluetooth IDs and GPS positions of a device and the meeting time are used to create a unique fingerprint of the geolocation.
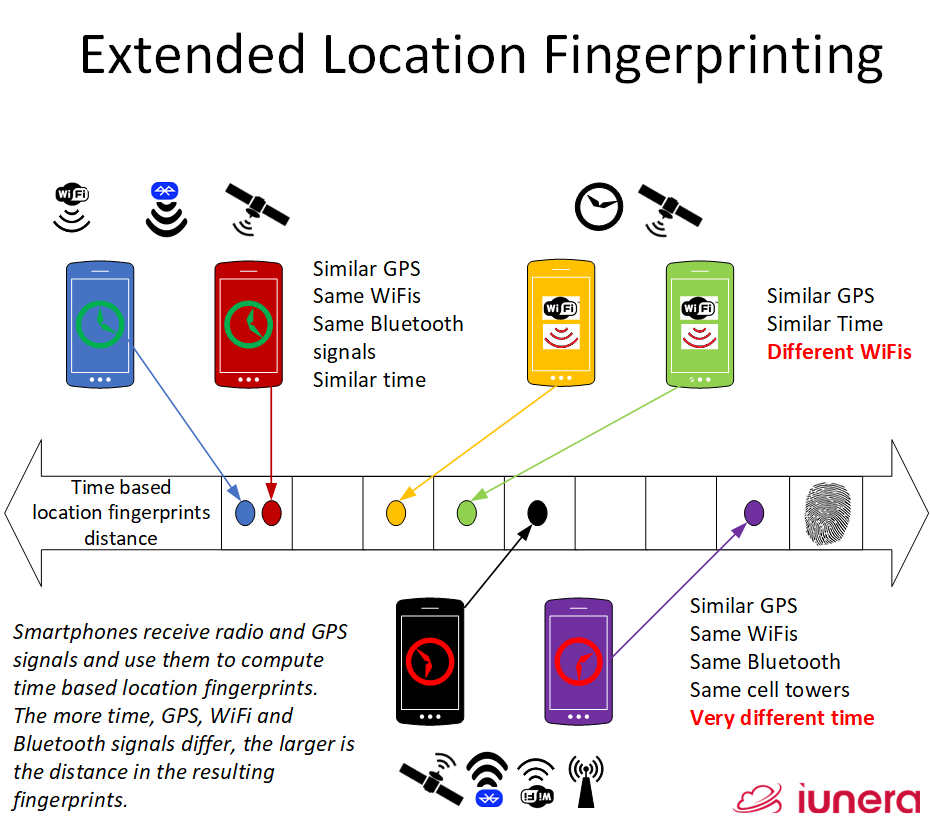
This fingerprint is generated by the use of a Local Senstive Hashing algorithms (or similar means) where a reconstruction of the original values is incredibly difficult.
The fingerprint distances can be computed and the extent of the distance of the fingerprint reflects the distance of the original values.
Only this fingerprint is sent to a Big Data infrastructure for matching distances. This way the original geoposition and other data is hidden from the server.
What is required to execute it?
A smartphone app, capturing the GPS, WiFi and Bluetooth signals and processing it all together into a location fingerprint.
A database will be on a server which matches the different fringerprints based on their distances.
How precise and privacy compliant is it?
With just a fingerprint a user shares a lot of information with a backend Big Data infrastructure.
Starting with a user identification and the knowlege of the server which other user matched or which other fingerprints have a low distance.
All in all, this method offers more privacy than complete extended location matching, but it is not as privacy compliant as a short distance Coronavirus tracking Bluetooth app.
Can it break infection chains?
It is possibile to find potentially infected people via matching. Therefore, it can help to break infection chains.
What is most likely not possible anymore by using fingerprint anonymisation is the following: One cannot directly learn anymore on which factors were most relevant for an infection, as well as to generate infection predictions.
Therefore, breaking the infection chain capabilities is immense, but not as advanced as complete Coronavirus geotracking.
Third party tracking
In another article, we discuss in detail what benefits and motivation for third party app data tracking are and how different variants of it can be implemented. Here we provide a brief overview about the essence.
Aside of a very own Coronavirus app, required Big Data for precise tracking can also be acquired from third party sources.
Why?
Motivating citizens to install an extra app for Coronavirus geotracking can be effortful and costly.
After all, around 60% of a country need to use an app to create a significant effect.
Even if one assumes that a Coronavirus tracking app could market itself and Coronavirus app installations cost below the normal market app install costs (1,22 to 4,08 USD) it can be a huge factor to advertise installations.
In addition to the installation costs there are also Coronavirus app development costs and development speed and maintence costs of an app which need to be considered.
Therefore, it can be an option to rely on data of apps who receive the necessary data from smartphones anyway. Such apps are social networking apps, ridesharing apps and also the operarating systems extensions of smartphones.
How it works
Operating systems of smartphones contain extensions to accelerate location determination. In order for them to work they send data on discovered Bluetooth and WiFi devices to a backend server and receive a location for that.
One can tap into this proccess and fork the data stream which comes from a smartphone and use it for Coronavirus location tracking and analysis.
Once an infection occurs the historic location of this user is then matched with the other users who have been at the same locations and the potential infected can be warned.
The same process with slight adjustments can also be implemented with applications which are widely distributed to gain location updates regularly.

What is required to do this?
The cooperation of a third party app or operating system vendor who captures location data naturally is needed.
The vendor needs to fork the Big Data stream which is then stored in a Time Series Database or similar.
In addition to the pure data, the app or operating system vendor should ask the user for their consent for location tracking in the Coronavirus case.
Implementation wise, the third party app or operating system needs an extension that an infected user can report an infection.
Once an infection is reported the backend need to be programmed to match potentially infected people with the help of Big Data systems.
How precise and privacy compliant is it?
This method can be as precise as a special geotracking app. The precision of this method will depend of the capabilities of the host app or system where the Big Data for analysis is taken from.
The privacy of this method is questionable. It is very easy for a user to answer a consent question if the data can be used for Coronvairus tracking, without understanding what is going on by using a long disclaimer.
If the user understands the extend as to which kind of data is used when giving consent with a short click can be questioned.
In addition, once such a method is rolled out the threat is that the temptation is there to not ask the users for consent once a Coronavirus outbreak grows stronger.
All in all, the Big Data analysis gives deep insights about a users behaviour and the consent from a user needs to be acquired in a honest and transparent way.
Can it break infection chains?
Like extended geotracking this method can break infection chains.
It is also possible to get second grade infections by matching potentially infected people with possible people they could have infected already.
Due to the possibility that the host smartphone application and also backend of an app or mobile operating system is already rolled out, there will very quickly be a huge user base.
Such a huge user base can help to track down infections and break infection chains much faster than custom developed apps where users need to be convinced to download and use the app.
Conclusion
We discussed different forms of how Big Data Analysis and smartphone Coronavirus apps can help stop the spreading speed of the Coronavirus.
Big Data Analysis of mobile provider reveals insights how movement and restriction and control oders can be analyzed better with additional data from cell towers.
Privacy compliant Bluetooth tracking apps make an immese amount of privacy for users possible, but require a large amount of citizens to download and use the app. Geotracking infection chains with privacy compliant Coronavirus Bluetooth apps is not possible due to the privacy approach.
Extended location tracking also requires an app, and it also makes it possible to track infection chains with geopositions through a central Big Data infrastucture based component. Ultimately, even possible infections of the second grade can be estimated. The price for this extended functionality is the lacking privacy for the users.
In order to enable more privacy in location based approaches, we described location fingerprinting where only a fingerprint of a location is shared with a Big Data infrastucture. A location fingerprint is computed by local sensitive hashing and therefore a Big Data infrastucture can compute distances but does not know the original ingredients of the fingerprint. The downside of this privacy addition is less accuracy and analysis capabilities at the server.
Lastly, we discussed the problem that own apps for Coronavirus tracking need over half of a countries population to work properly. We illustrated how difficult or expensive it could be to get this amount of required installations.
We showed a concept of how data from mobile operating systems or third party apps can be feed into a Big Data Coronavirus tracking and analysis backend. For this concept to work, users consent is required for the additional data use.
The downside is this third party data analysis approach is the least transparent method for the users. On the contrary, at the same time also the most feasible method with the highest practability and fastest implementation time.
In addition, with a consent question in an already trusted app, it is very likely that more people will participate in giving their data than in installing a new and untrusted Coronavirus app.
Comparison and verdict
In order to give a better overview, we sum up advantages and disadvantages of Big Data Analysis, Coronavirus apps and privacy in the following comparison matrix:
Mobile provider tracking | Privacy Bluetooth tracking | Extended location tracking | Location fingerprint tracking | Third party app/OS tracking | |
---|---|---|---|---|---|
Own app required | No | Yes | Yes | Yes | No |
Privacy | XXX | XXX | X | XX | X |
Precision | X | XX | XXX | XX | XXX |
Infection chain analysis | No | X | XXX | XX | XXXX (extreme through many users) |
All approaches have their pros and cons. In short, the more privacy and transparence, the less tracking and functional possibilities are there.
The less privacy and transparency the more analysis and precison is possible.
Ultimately, it is up to the different countries and politicians on how much of privacy is forsaked to contain the speed of the virus spread with advanced analysis methods.
Update (29th April 2021): Check out our latest project, Fahrbar!
Get in touch with us
If you are interested in Fahrbar or want to find out how we can help you leverage your data