I have a seashell on my desk. It serves as a personal reminder to always think out of the box and widen my viewpoint when solving complex Big Data Analysis challenges.
In this article you learn:
- Why Data Scientists carry risks like fishermen?
- What is Chicken-Egg problem of Data Science?
- How does the Chinese fishing nets in India get lifted up?
Each time I look at the shell, I’m brought back to the day I found it; it was the most fascinating and intriguing art condensed to extract value from mass fishing with the least effort. This was pretty much where I found it:
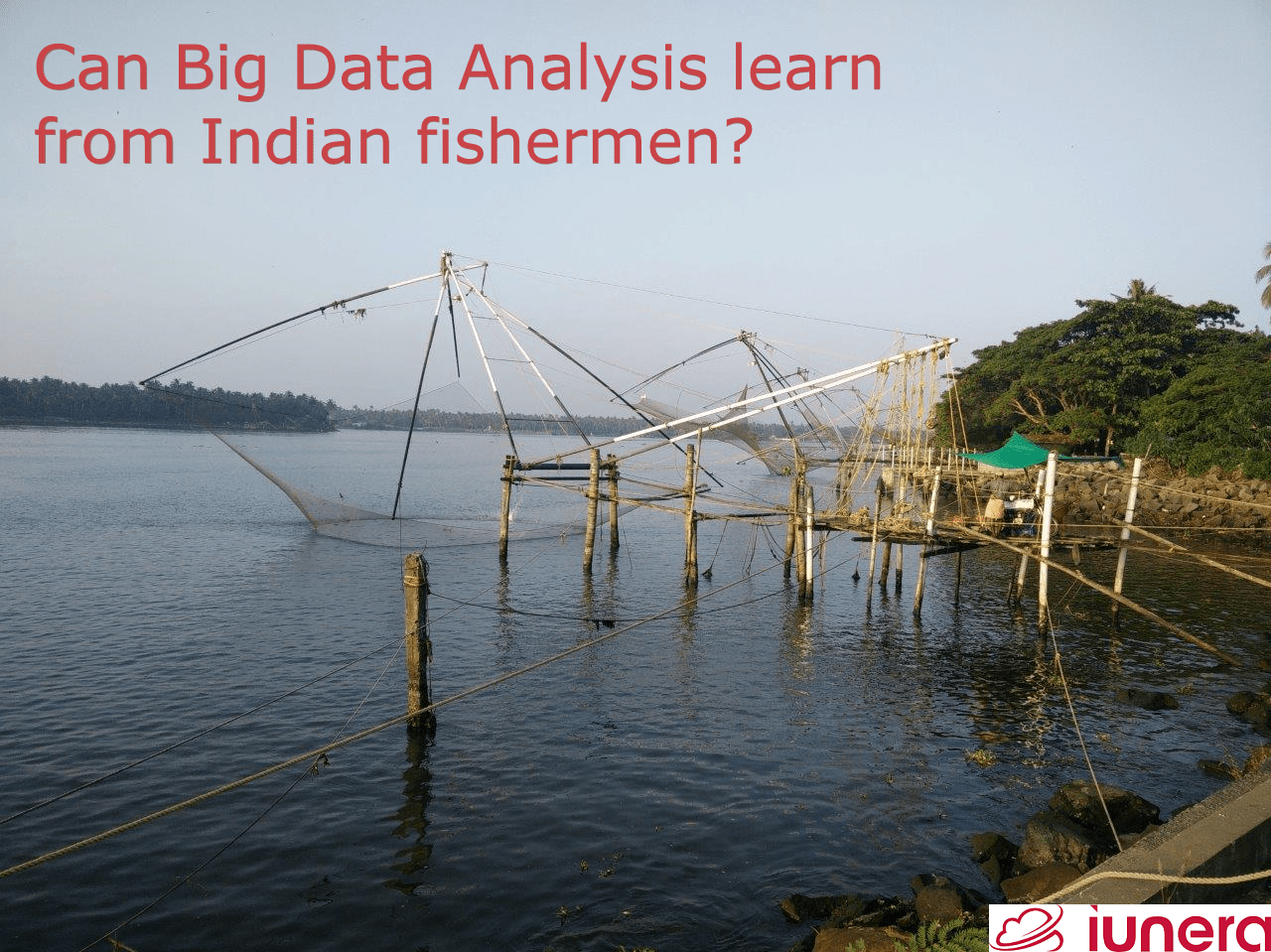
- Big Data Science in big oceans – the correlation
- How does lift net architecture relate to Big Data Analysis and Data Lakes?
- How data Scientists extract value from Big Data tools
- The chicken-and-egg problem of Data Science
- Conclusion
- Sum-Up FAQ
- Update (29th April 2021): Check out our latest project, Fahrbar!
Big Data Science in big oceans – the correlation
Procuring my very own shell was similar to uncovering and appreciating the value of Big Data Analysis; this shell originated from an ocean of artifacts, and the value of Big Data Ocean is an ocean of data.
Data scientists cast their own lift nets just like these fishermen with their large nets, fish for information in the data oceans, and once they find a valuable artifact, they proudly present them to their fellow peers.
Perhaps the difference between these two activities are that the ‘oceans’ which these Data Scientists cast their nets in are massive; I’d personally use trawling nets for my endeavours.
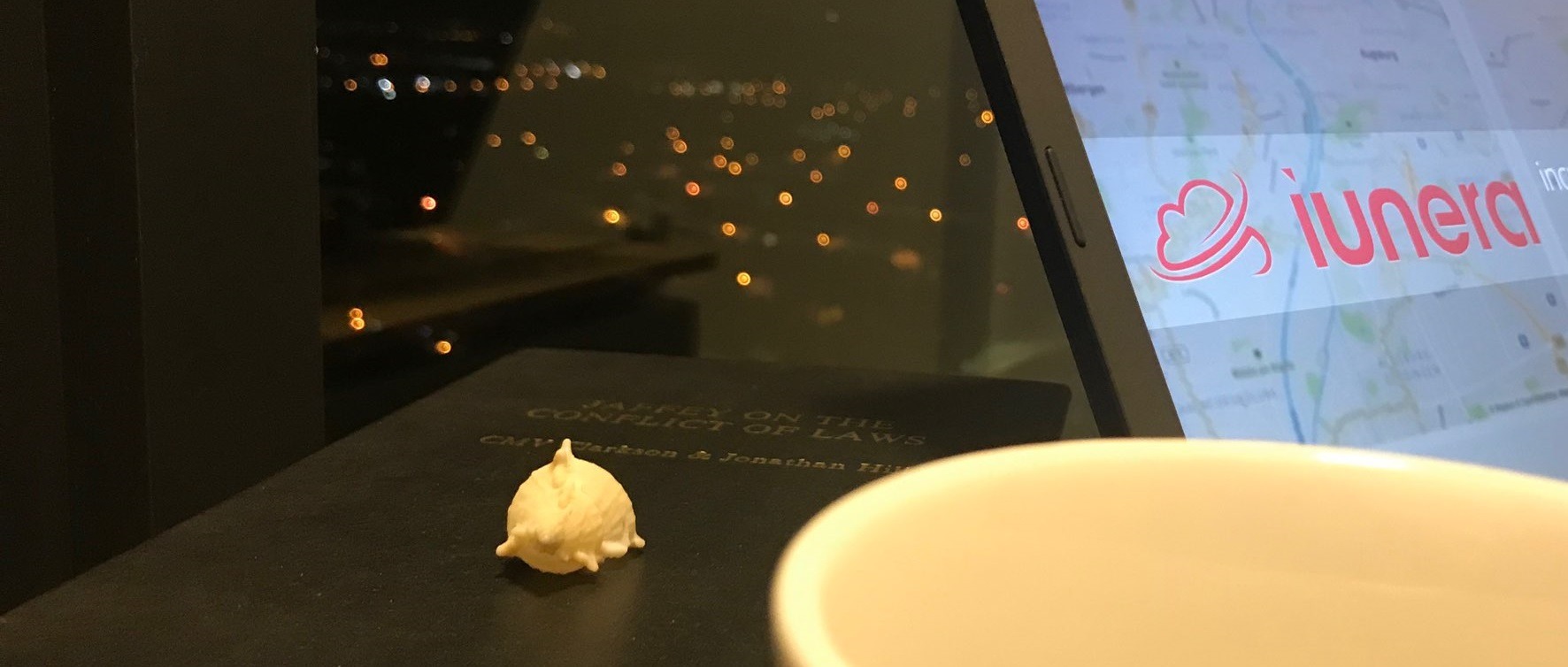
How does lift net architecture relate to Big Data Analysis and Data Lakes?
Finding the correlation with the ocean was my a-ha moment for Big Data Analysis. I was near Cochin, India when I saw these lift nets. I was fascinated by it and looked and watched these fishermen work with them.
While I studied how this immense fishing tool worked, a fisherman gestured me to come over and showed me how it works. He then gifted me my first shell.
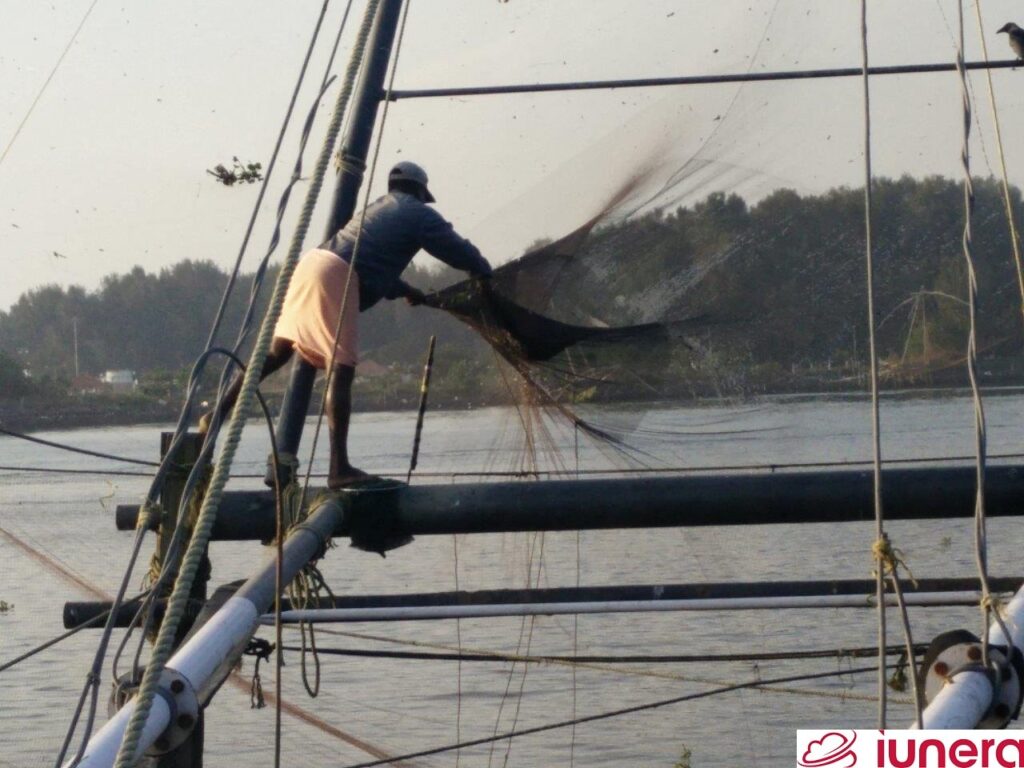
In a (nut)shell, this lift net is an apparatus to assist in fishing, where a huge net is lowered into the water and then pulled up by a lever attached to a rope wound around a motorbike. Once lifted, the content of the net becomes visible to the fisherman. See this technology yourself in action:
This is not so different to a Big Data Analysis setup; we collect information out of various systems and make it accessible for Big Data Analysis. All information is collected, provided and automated via Big Data Analysis tools.
Ultimately, the net which extracts the information is then given access to the Data Scientists who examines and investigates the Data Lake for Big Data Analysis.
In order to visualise this better, I have made the following picture. Here, you see the various data sources which are integrated to do Big Data Analysis.
The data is then structured, cleaned and stored into models, enlisted in a repository and flagged as master or transactional data for analysis. Data Scientists then use this information to examine and investigate thoroughly to make their catch worthwhile.
From this we can make the following conclusions:
- Using the right tool is essential to fish in the Big Data Ocean for Big Data Analysis
- Using automated processes and tools enables Data Science talents to mine and do Big Data Analysis easily
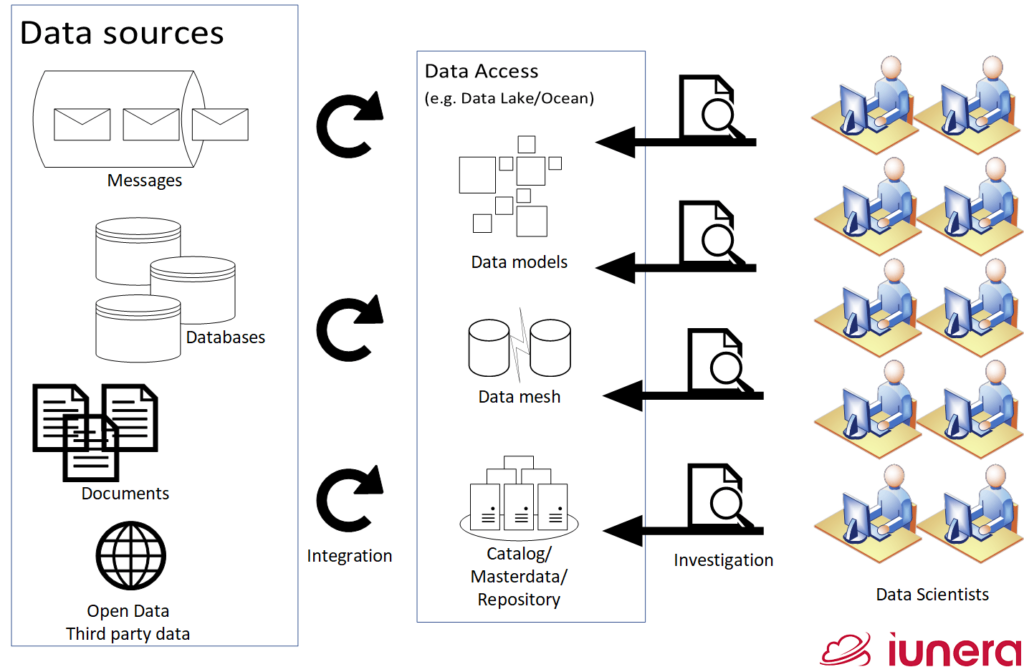
How data Scientists extract value from Big Data tools
In Data Science, we have a Big Data Ocean full of velocity, variety and massive volume that one needs to access to mine facts from it.
Experienced Data Architects and experts will also determine the positions to install the right tools to extract and integrate the most amount of information. Subsequently, Data Engineers then construct Big Data Analysis tools like the lift net to integrate and access the information stored in the ocean.
Despite building these infrastructures with a limited supply of talent, Data Engineers construct automated processes to extract, monitor, mesh and model data integration and provision the necessary tooling to investigate the data.
Once all necessary tools are installed, Data Scientists can operate these ‘fishing devices’ and lift out the Big Data and investigate its results, the content.
At most times, the information is inadequate, and some additional information needs to be extracted. Once this picture is complete, it would be enough for the catch to be presented to influence business decisions.
We can take from this the following lessons:
- Ensure your tooling is reliable and already in production
- Establish standard procedures for how value should be extracted from the Big Data Ocean
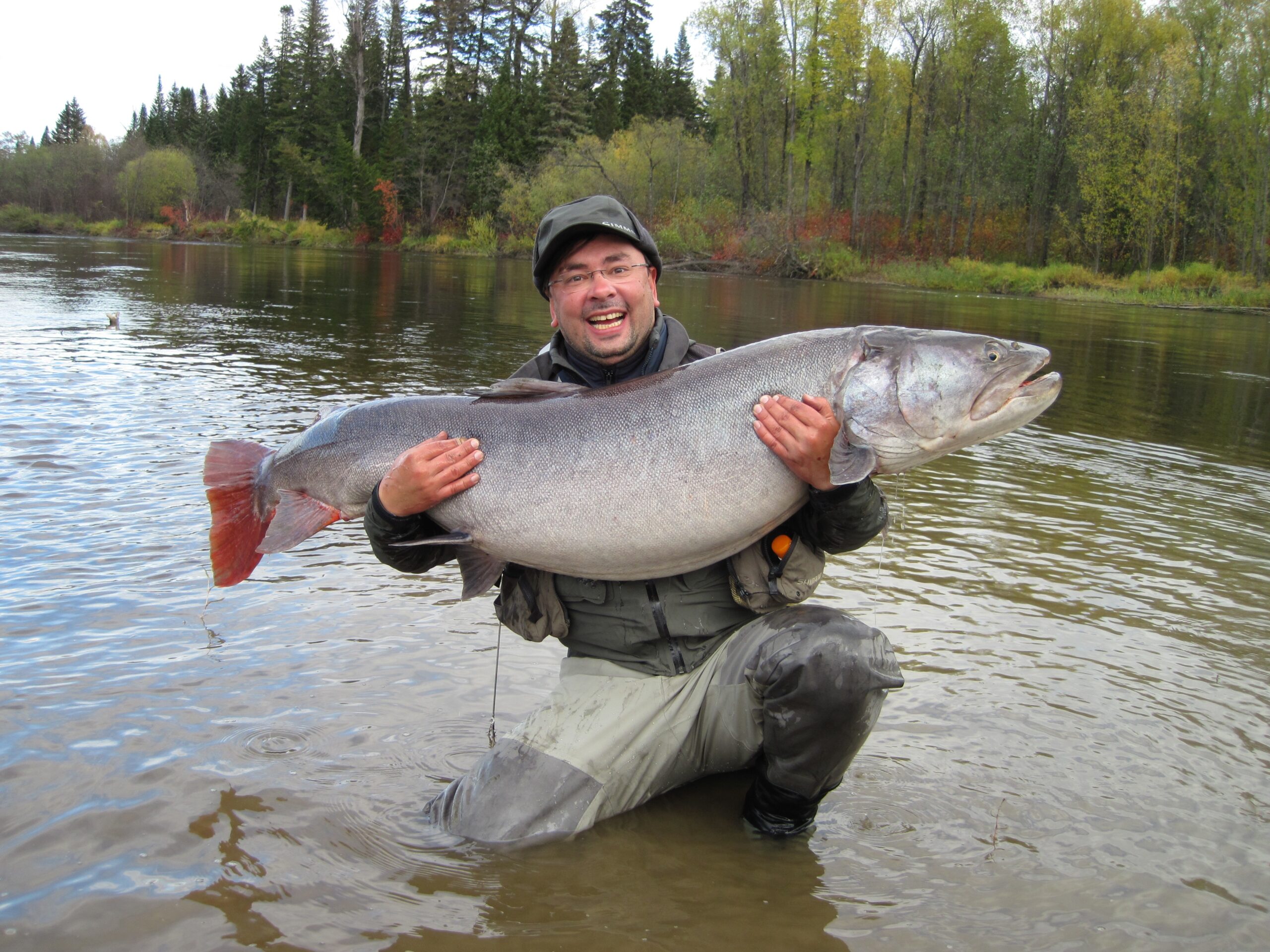
Source: https://pxhere.com/fr/photo/1278316
The chicken-and-egg problem of Data Science
Like a fisherman, the Data Scientist, the Data Engineer and the Data Architect together would not know if they will find value in the Data Ocean. All the Data Engineers’ tooling for Big Data and the Data Scientist‘s exploration is just done on an assumption that they likely will find value. This makes their mutual trust with each other an essential value to share in this line of job.
Furthermore, this uncertainty makes it hard to start Data Science projects and scale them; first, there needs to be evidence that there is value to get full commitment, sponsorship and unswerving support from business departments. Without evidence and full management commitment, it is hard to get sponsors and support.
This ultimately is a chicken-and-egg problem. As it is for the sea, the problem of the fishermen there was solved centuries ago: any fisherman carries the risk of having a catch or not. Hence, the fisherman takes care of his work equipment very carefully and trains himself to utilise his tools perfectly.
The same logic applies to Data Scientists and engineers: they learn and sharpen their skills step by step to deliver quick turnovers and ultimately Big Data Analysis results once data is available.
Ultimately, there is no certainty for a catch in each execution. The ultimate goal of a project must be to provide the initial value to overcome the chicken-and-egg problem. This is the crucial first step to advance tooling, procure results and to scale.
In order to get a project running, we learn from the fishermen’s craft:
- To provide the first value in the form of Big Data Analysis results as quickly as possible as it has a real impact on the sponsors
- Big Data Science Talent need to enhance their skills and tools continuously to ensure success quickly when a new project gets started
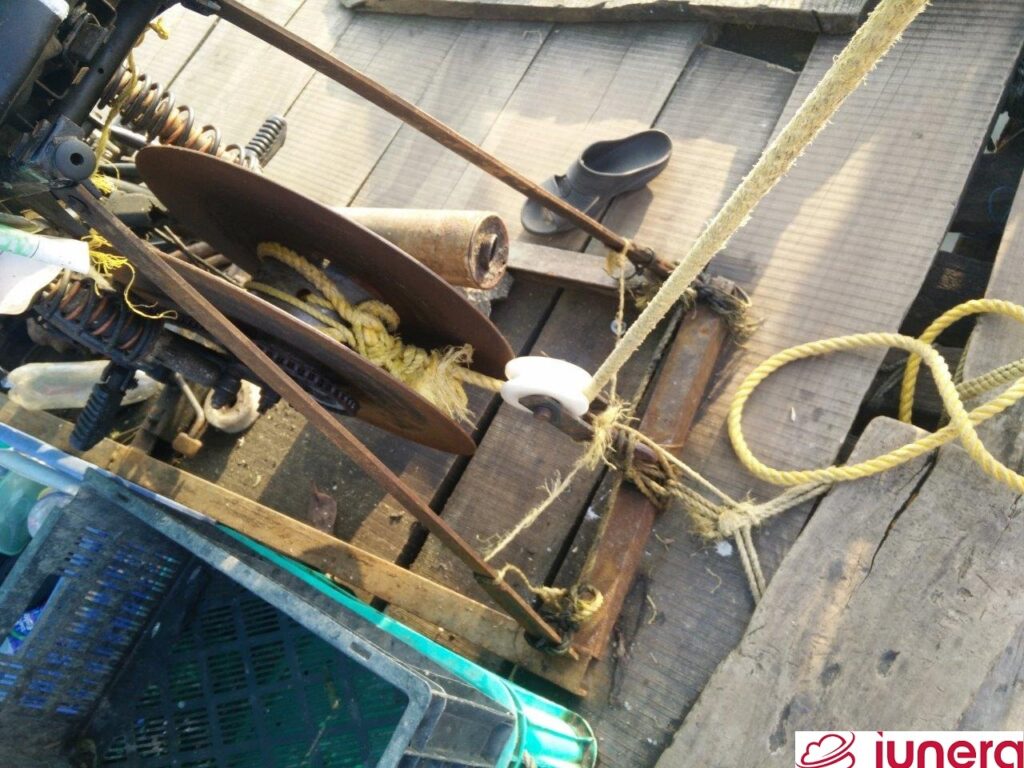
Conclusion
I believe that the analogy between the fishermen of Kerala and Big Data Analysis is an interesting one. We have discussed several similarities and saw different challenges and obstacles Big Data Science teams need to overcome.
Thereby, we found that the tooling and processes of the fisherman can be inspiring for our Big Data Science projects. Here’s what our key learnings are:
- Automated Big Data Tools and processes are essential to run in production
- Providing first productive value as quickly as possible as it has a real impact to this ecosystem
- Big Data Science Talent need to enhance their skills and tools continuously to ensure a catch quickly, when a new project comes in
Sum-Up FAQ
Find below the most important questions and answers of this article.
What project risks do Big Data Scientists carry naturally?
The explorative work of the Data Scientist is just done on an assumption that they likely will find value. There is no certainty for a catch in each execution.
What is the Chicken-Egg problem of Data Science?
There needs to be evidence that there is value to get the full commitment, sponsorship and unswerving support from business departments. Without evidence and full management commitment, it is hard to get sponsors and support. Data-driven projects need results, but in order to generate this sponsorship is needed.
How can Data Science project results overcome the chick-egg problem?
They need to provide the first value in the form of Big Data Analysis results as quickly as possible as it has a real impact on the sponsors.
What is the role of tooling in Data Science projects?
Automated Big Data Tools and defined processes are essential to run in production. This is necessary to ensure testability and reliability.
Update (29th April 2021): Check out our latest project, Fahrbar!
Get in touch with us
If you are interested in Fahrbar or want to find out how we can help you leverage your data